Introduction
In empirical finance and many other domains, linear regression and the closely related linear prediction theory are commonly used statistical methods. Because of the wide range of applications, basic linear regression courses normally concentrate on the mathematically simplest scenario, which can be used in a variety of other applications.
Ordinary least squares (OLS)
A linear regression model relates the output (or response)
where the
To fit a regression model to the observed data, the method of least squares chooses
Setting to 0 the partial derivative of RSS with respect to
The vector of least squares estimates of the
Using this matrix notation, RSS can be written as
Statistical Properties of OLS Estimates
are nonrandom constants and has full rank p, where . are unobserved random disturbances with and for are independent , where denotes the normal distribution with mean and variance .
Case Study
We illustrate the application of this methods in a case study that relates the daily log returns of the stock of Microsoft Corporation to those of several computer and software companies.
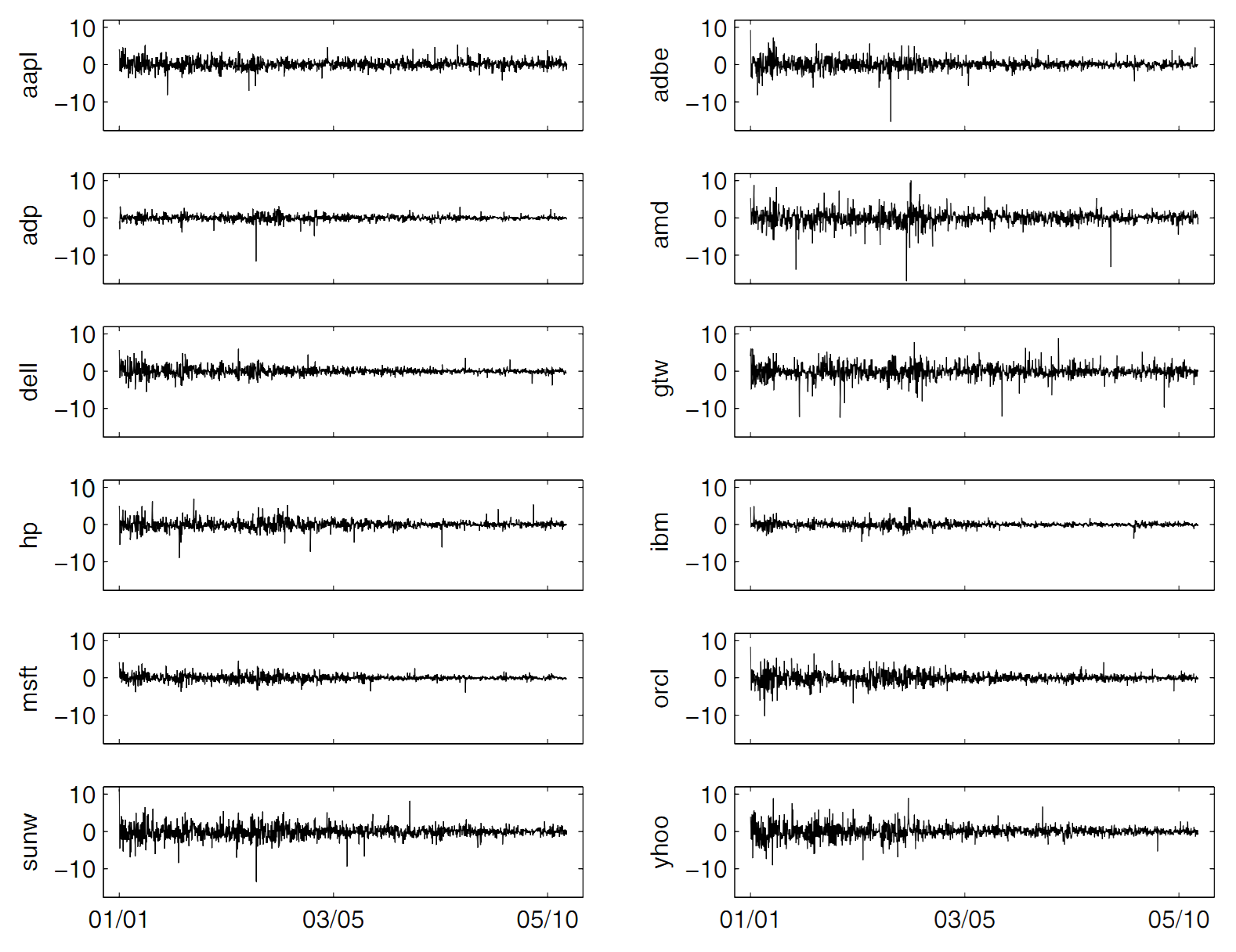
Starting with the full model, we find that the stocks hp and sunw, with relatively small partial F-statistics, are not significant at the 5% significance level. If we set the cutoff value at
Regression coefficients of the full model.
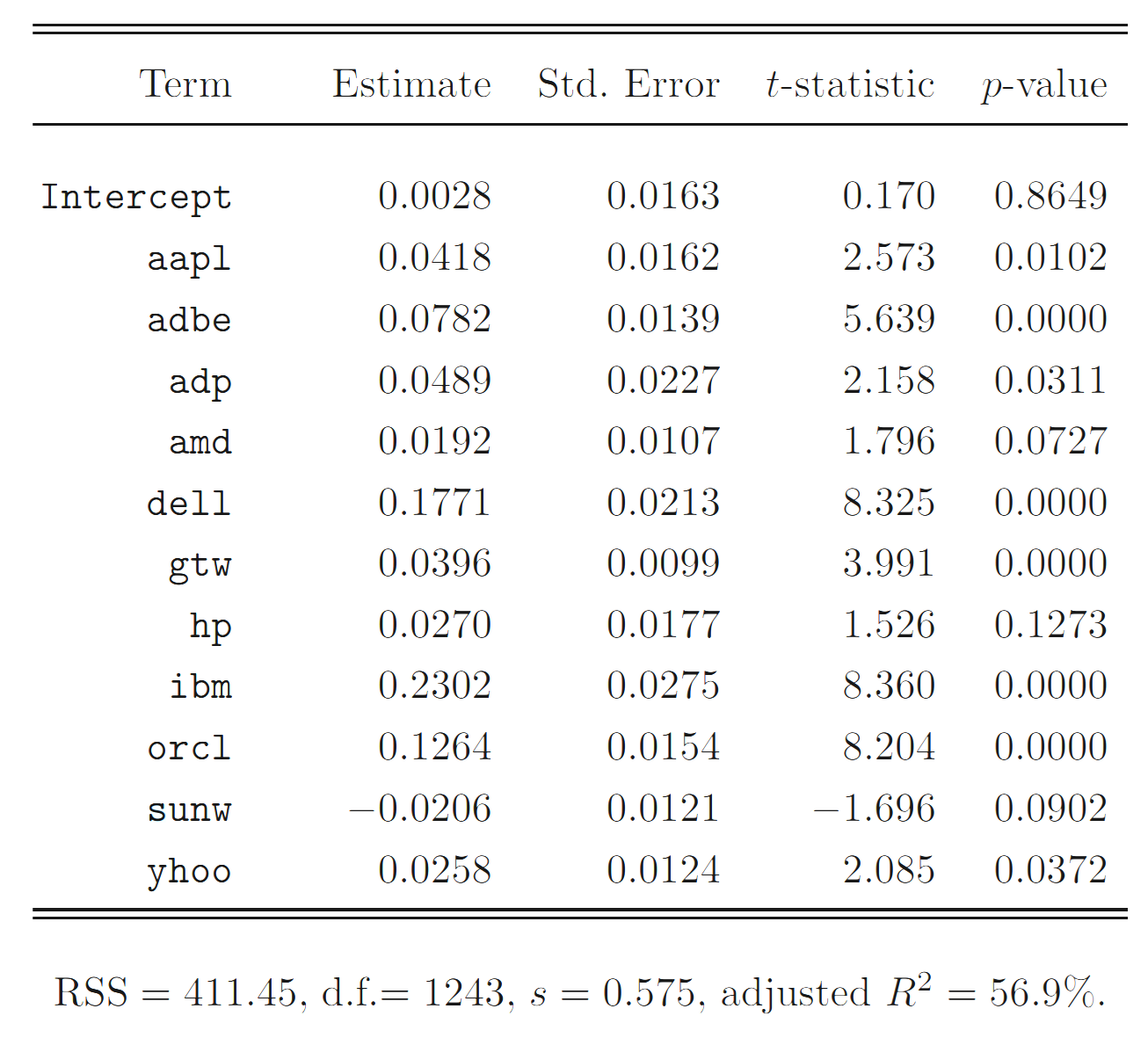
Regression coefficients of the selected regression model.
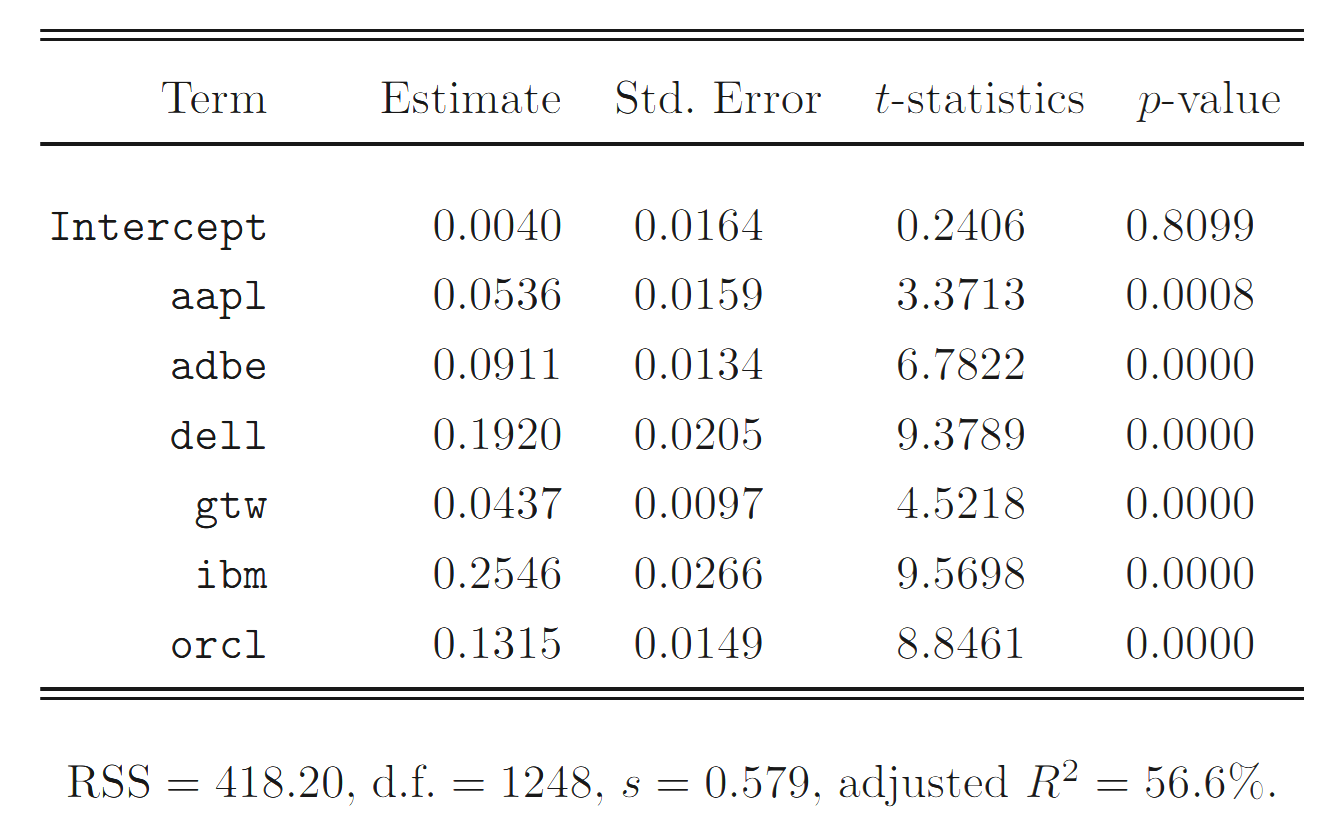
The selected model shows that, in the collection of stocks we studied, the msft daily log return is strongly influenced by those of its competitors.
Conclusion
The importance of regression analysis lies in the fact that it provides a powerful statistical method that allows a business to examine the relationship between two or more variables of interest.
References
- Lai and Xing, Statistical Models and Methods for Financial Markets (2008)